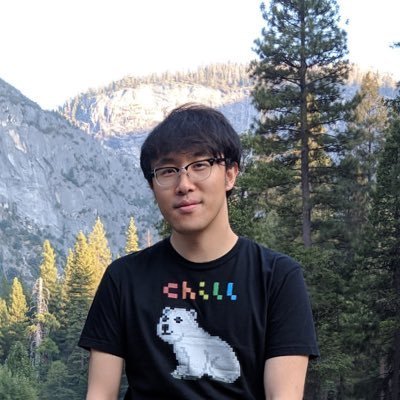
Saining Xie
@sainingxie
Followers
20K
Following
5K
Media
55
Statuses
495
researcher in #deeplearning #computervision | assistant professor at @NYU_Courant @nyuniversity | previous: research scientist @metaai (FAIR) @UCSanDiego
Joined July 2020
During my internship at DeepMind, Demis met with all the interns. When asked about the company’s goal, I vividly remember him saying, “winning *multiple* Nobel prizes.” I was shocked at the time, but now, just 7 years later, part of that mission is already accomplished. Eager to.
BREAKING NEWS.The Royal Swedish Academy of Sciences has decided to award the 2024 #NobelPrize in Chemistry with one half to David Baker “for computational protein design” and the other half jointly to Demis Hassabis and John M. Jumper “for protein structure prediction.”
10
112
2K
A new chapter of my professional life! After 4 incredible years at FAIR and living in the bay, I’m moving to NYC! I’ll be joining @NYU_Courant CS @NYUniversity @CILVRatNYU as an Assistant Professor the upcoming Jan. Looking for students/postdocs to join me on this new adventure!!
53
23
619
Wow, Deeply Supervised Nets received the Test of Time award at @aistats_conf 2025! It was the very first paper I submitted during my PhD. Fun fact: the paper was originally rejected by NeurIPS with scores of 8/8/7 (yes, that pain stuck with me. maybe now I can finally let it.
The #AISTATS 2025 Test of Time Award goes to . 🥁 . Chen-Yu Lee, Saining Xie, Patrick Gallagher, Zhengyou Zhang, Zhuowen Tu, for "Deeply Supervised Nets"! Congratulations!
33
43
508
When I first saw diffusion models, I was blown away by how naturally they scale during inference: you train them with fixed flops, but during test time, you can ramp it up by like 1,000x. This was way before it became a big deal with o1. But honestly, the scaling isn’t that.
Inference-time scaling for LLMs drastically improves the model's ability in many perspectives, but what about diffusion models?.In our latest study—Inference-Time Scaling for Diffusion Models beyond Scaling Denoising Steps—we reframe inference-time scaling as a search problem
9
69
478
Diffusion Transformer architecture + Flow Matching / Stochastic Interpolants objective? Great work and looking forward to the technical report!. In SiT ( we have also studied this new design space under class conditional generation (though on a much.
Announcing Stable Diffusion 3, our most capable text-to-image model, utilizing a diffusion transformer architecture for greatly improved performance in multi-subject prompts, image quality, and spelling abilities. Today, we are opening the waitlist for early preview. This phase
5
48
350
i think this just shows the input image passes through semantic encoders instead of VQ; they're aligned with the LLM and grasp image content well (super important for editing) but may not perfectly reconstruct original pixels (due to capacity limits / # image tokens).
ChatGPT insists on swapping out real faces for fake ones, when requested to generate an identical image to the input. Below (input, output)
16
25
386
thought experiment: ViTs work great for 224^2 images, but what if you had a 1 million^2 pixel one? You'd either use conv, or you patchify and process each with a ViT using shared weights—essentially conv. a moment I realize convnet isn't an architecture; it's a way of thinking.
A short post on the best architectures for real-time image and video processing. TL;DR: use convolutions with stride or pooling at the low levels, and stick self-attention circuits at higher levels, where feature vectors represent objects. PS: ready to bet that Tesla FSD uses.
14
26
339
Diffusion Transformer (DiT) just got an upgrade! .Same backbone but better quality, speed and flexibility. And we achieved this by. moving beyond standard diffusion and exploring a broader design space with interpolants!. Introducing SiT -- Scalable Interpolant Transformers!.
NYU presents SiT. Exploring Flow and Diffusion-based Generative Models with Scalable Interpolant Transformers. paper page: present Scalable Interpolant Transformers (SiT), a family of generative models built on the backbone of Diffusion Transformers
5
40
300
Nothing ever happens… until it does! . Just saw JanusFlow by @deepseek_ai uses REPA for training and shows some solid improvements: .
@cloneofsimo hmm I’ve got a bunch of independent data points now showing that REPA helps with big text-to-image models too! Let’s give it a little more time before saying nothing ever happens—I’m sure it won’t be long :).
4
31
261
Our take on a 4o-style AR + diffusion unified model: Transferring knowledge from an AR LLM to generation is easier than expected--you don't even need to touch the LLM. The right bridge between output modalities can unlock cool capabilities like knowledge-augmented generation!.
We find training unified multimodal understanding and generation models is so easy, you do not need to tune MLLMs at all. MLLM's knowledge/reasoning/in-context learning can be transferred from multimodal understanding (text output) to generation (pixel output) even it is FROZEN!
4
29
262
About two years ago, we started building V* to bring visual search into a multimodal LLM and show that it's a key part of how these models can understand the world. I still remember talking with my friend @bowenc0221 and @_alex_kirillov_ about why this.
🔍Introducing V*: exploring guided visual search in multimodal LLMs. MLLMs like GPT4V & LLaVA are amazing, but one concern that keeps me up at night: the (frozen) visual encoder typically extracts global image tokens *only once*, regardless of resolution or scene complexity (1/n)
2
27
250
In Cambrian-1, we found that vision SSL representations usually lagged behind language-supervised ones -- but once the data gap is closed and scaling kicks in, performance catches up. We’ve tried scaling SSL before, but this is the first time I’ve seen real signal: SSL adapts to.
Can visual SSL match CLIP on VQA?. Yes! We show with controlled experiments that visual SSL can be competitive even on OCR/Chart VQA, as demonstrated by our new Web-SSL model family (1B-7B params) which is trained purely on web images – without any language supervision.
2
51
246
Some further thoughts on the idea of "thinking with images":. 1) zero-shot tool use is limited -- you can’t just call an object detector to do visual search. That’s why approaches like VisProg/ViperGPT/Visual-sketchpad will not generalize or scale well. 2) visual search needs to
@WenhuChen @WeijiaShi2 at a glance, this is quite diff than what we did fwiw. the behaviors you see in o3 and o4-mini are all emergent from large-scale RL. we just give them access to python and the ability to manipulate images, the rest is up to the model.
5
32
242
#shamelessplug DiT shines in Sora. Our team at NYU has recently released a new DiT model, called SiT. It has exactly the same architecture, but offers enhanced performance and faster convergence. Super curious about its performance on video generation too! (n/n).
4
21
229
I like pretty pictures but one thing I like more about diffusion models is how they open up new doors for (useful) analysis-by-synthesis approaches. More and more research is showing that (pre-trained) diffusion models are pretty good feature extractors too. This empirical study.
Meta presents Deconstructing Denoising Diffusion Models for Self-Supervised Learning. paper page: examine the representation learning abilities of Denoising Diffusion Models (DDM) that were originally purposed for image generation. Our philosophy is to
5
26
214
Almost every deep learning model for 3D recognition has been *trained from scratch*. In our #ECCV2020 spotlight paper, we propose 👉PointContrast👈, an unsupervised pre-training framework that boosts performance on 6 different 3D point cloud benchmarks.
3
40
201
@jbhuang0604 there's no true self-supervised learning in text - it's (strongly) supervised learning.
7
4
152
Really enjoyed working on this project; some thoughts on why I believe combining the creative freedom of generative models with the precision of the 3D graphics pipeline could be the future. (1/n)🧵.
Intel and NYU present Image Sculpting. Precise Object Editing with 3D Geometry Control. paper page: present Image Sculpting, a new framework for editing 2D images by incorporating tools from 3D geometry and graphics. This approach differs markedly from
3
17
142
The pre-trained, frozen VAE in DiT is massive (much higher flops than the transformer itself!!). Do you have to freeze it? What if you could leverage that capacity through e2e training? .It turns out it doesn't play well with diffusion loss -- but with REPA, you can make it work!.
Can we optimize both the VAE tokenizer and diffusion model together in an end-to-end manner? Short Answer: Yes. 🚨 Introducing REPA-E: the first end-to-end tuning approach for jointly optimizing both the VAE and the latent diffusion model using REPA loss 🚨. Key Idea:.🧠
2
16
142
Check out the latest paper from @TongPetersb on grokking both visual understanding and generation abilities through (modest scale) instruction tuning. The data composition reveals both asymmetry and prioritization: we represent to understand, and we understand to create.
How far is an LLM from not only understanding but also generating visually?. Not very far!. Introducing MetaMorph---a multimodal understanding and generation model. In MetaMorph, understanding and generation benefit each other. Very moderate generation data is needed to elicit
1
11
113
It was a blast seeing everyone at nyu and getting to learn about all the cool work. this is why nyc (and surroundings) are a great place for computer vision 😊.
Fresh memories from 🗽NYC Vision Day🗽 hosted at NYU yesterday, April 1st (. Grateful to the organizers (David Fouhey, @jiadeng, @orussakovsky, @Jimantha, @cvondrick @sainingxie) for setting up such an amazing event to bring the vision community together!
3
3
113
team industry -1, team academia +1 🎉😉. students, here’s your chance to join an amazing lab ⬇️.
Excited to share that I will be joining Princeton Computer Science @PrincetonCS as an Assistant Professor in September 2025!. I'm looking for students to join me. If you are interested in working with me on VLMs, LLMs, deep learning (vision/LLM) architectures, data, training,.
1
1
104
Check out our paper for more details: Visit our project page: Code and models are also available: Big shoutout to our intern @penghaowu2 for the heroic work! (n/n).
4
17
98
Unsung hero for academia.
everybody talks big game about democratizing AI, but today I'm super grateful that @GoogleColab gives free TPU + GPU instances. Makes it possible for a lot of students to learn about ML without spending a few thousand dollars building a deep learning PC.
0
3
92
Recently open-sourced projects from @TongPetersb, @DavidJFan, and the team at Meta FAIR. MetaMorph (training code and model weights): Web-SSL (model weights for Web-DINO and Web-MAE). FAIR's still leading the way in open research.
We are open-sourcing all the models in Web-SSL, from ViT-L to ViT-7B! .It was super fun to train and play with these massive ViTs. Models: Github: Huge credit to @DavidJFan for putting these models together!.
1
13
87
Excited to present in the upcoming tutorials/workshops/posters and reconnect with old friends at #ECCV2024 Milano!.Sunday AM (29th): Tutorial on Large Multimodal Foundation Models (.Sunday PM (29th): 2nd OmniLabel Workshop on Enabling Complex Perception.
0
8
82
Arrived in Vancouver for #NeurIPS2024 now! Don’t miss @_ellisbrown and @TongPetersb’s talk about Cambrian-1—I’ll be there and at the poster too. Excited to connect with you all!.
Heading to #NeurIPS2024 to present Cambrian-1 w/ @TongPetersb! Catch our oral presentation Friday @ 10am (Oral 5C) and our poster afterwards until 2pm (#3700 in East Hall A-C) 🪼🎉
0
4
79
As @ylecun often points out, relying solely on the "rendering" loss isn't enough. If your focus is just on reconstructing nice-looking pixels, there's no way to filter out irrelevant details from the input — which is key to learning robust representations. Looks like even if your.
1
4
73
Jiatao is such an AI polymath, with amazing knowledge and experience across so many areas🤯! Every time I chat research with him, I come away learning so much. You should definitely apply to his lab—it’d be an incredible experience!.
Life update: Excited to share that I will be joining @CIS_Penn @PennEngineers as an Assistant Professor in Fall 2025!🤯. I’m also seeking multiple PhD students passionate about Generative Intelligence and leveraging it to empower AI agents to interact with the Physical World🌟
1
0
68
Looking forward to attending in person tomorrow!.
A reminder that our Transformers for Vision workshop in #CVPR22 is happening this Sunday, June 19th at 7:50am CST in Great Hall D (and also on Zoom). We have an amazing speaker lineup, great panelists, and excellent paper sessions. Looking forward to seeing everybody!
2
6
64
Excited to see more open multimodal LLMs!.Also quite impressive vision-centric performance on e.g. MMVP ( with Gemma-2B.
We release PaliGemma. I'll keep it short, still on vacation:. - sota open base VLM designed to transfer quickly, easily, and strongly to a wide range of tasks.- Also does detection and segmentation.- We provide lots of examples.- Meaty tech report later!.
2
7
64
not vibe/value alignment but “reality alignment”: we could let the model imagine in the multi-universe, then align it to earth in post-training. @giffmana you might find this interesting too then:
This paper is interestingly thought- provoking for me. There is a chance, that it's easier to "align t2i model with real physics" in post-training. And let it learn to generate whatever (physically implausible) combinations in pretrain. As opposed to trying hard to come up with.
2
12
64
Welcome Gautam! So excited to have you join us🥳👏!!. Thinking about a PhD in AI? @NYU_Courant is a great place, and so is NYC. Apply before the deadline!.
Excited to announce that I'm joining NYU Courant (@NYU_Courant) CS as an Assistant Professor in Fall 2025 (~1 year from now). If you wish to work with me as a PhD student in theory/empirics of robustness/privacy in stats/ML (or related topics), apply to Courant CS by Dec 12! 1/n
0
1
54
We have the model & a local gradio demo that you can download & play with:
This new V* (guided visual search) model & paper is actually a big deal in my opinion. GPT-4V could *not* solve this google recaptcha I had been testing. But now. with the help of the guided V* model it could find the final traffic light
0
5
55
Great to see this got a spotlight at ICLR & the community has welcomed a "pure data" paper with open arms. Congrats @Hu_Hsu!.
This difference in data sources and filters is highlighted in our "Demystifying CLIP Data" ( paper. Instead of viewing it as a fresh "MetaCLIP" model family, think of it as a "manual for building CLIP from the ground up".
0
2
54
The true golden age is one of exploration, not exploitation.
This thread by @scott_e_reed, one of the best deep learning researchers in the world, summarises well what many experienced working for industrial AI .labs over the last two years: . 1. Winner take all politics.2. An erosion of our ability to innovate .3. An erosion of our belief.
0
3
50
The project was led by our amazing PhD student @TongPetersb at @NYU_Courant, and it was a great collaboration with folks at NYU and Berkeley!. ArXiv: Blogpost: Code:
3
7
50
imagination is generative; control is 3D.
Today we're sharing our first research update @theworldlabs -- a generative model of 3D worlds!. I'm super proud of what the team has achieved so far, and can't wait to see what comes next. Lifting GenAI to 3D will change the way we make media, from movies to games and more!.
1
4
49
Congrats @rob_fergus ! Big win for FAIR.
1/ Excited to share that I’m taking on the role of leading Fundamental AI Research (FAIR) at Meta. Huge thanks to Joelle for everything. Look forward to working closely again with Yann & team.
1
2
44
a fun collaboration with the system group at nyu. through sparse all2all comm; dynamic load balancing and large batch hyperparameter scaling rule, now you can finally train your large 3dgs on many gpus🔥without any loss in quality. led by @zhaohexu2001 haoyang & @fred_lu_443.
On Scaling Up 3D Gaussian Splatting Training.Project: Code (Apache 2.0): => Parallelize training over multiple GPUs. Make sure to checkout the project page, it is awesome! .Method ⬇️ 1 | 2
0
6
43
How many labels do we need to train an instance segmentation model for 3D scenes? It turns out not too many! With the help of our new pre-training method Contrastive Scene Contexts, only 20 annotated points per scene are good enough to produce high quality results on ScanNet!.
Sharing our new work Contrastive Scene Contexts, a new pre-training method for data-efficient learning in 3D. New ScanNet benchmark coming up soon!. Project: Paper: (w/ BenGraham @MattNiessner @sainingxie).
0
4
40
This is mind blowing 🤯 congrats @billpeeb and the team.
Excited to share what @billpeeb @_tim_brooks and my team has been working on for the past year! Our text-to-video model Sora can generate videos of complex scenes up to a minute long. We're excited about making this step toward AI that can reason about the world like we do.
0
0
39
I'm delighted to see how the fun brainstorming with @drfeifei on spatial intelligence this year have evolved into an amazing collaboration between NYU, Yale, and Stanford. A huge shoutout to @jihanyang13, @shushengyang, @AnjaliWGupta, and @rilyn_han for leading this effort! If.
2
1
39
since many of you are curious -- the video was done by just me and students using tools like @dalle_openai @pika_labs @capcutapp @googleearth, music @blurofficial parklife (1994) -- we are ai researchers, and we are also ai users.
2
0
38
If you're interested in an internship at Google Research focusing on video generation🎞️, Xuan is definitely the person to talk to!.
We are hiring PhD interns to work on video generation research at Google Research US. Please reach out to xuanyang@google.com if you are interested.
0
3
35
NYU Courant is looking for motivated First Years and Sophomores of all backgrounds to join a 6 week summer program in AI research! Expenses paid plus stipend.
Applications are open for the Summer 2023 Pathways to AI Program!. We are seeking first and second-year undergraduates pursuing careers in AI research. Students from underrepresented groups are especially encouraged to learn more and apply by Feb. 1st:
1
9
36
Finally, we tried to recreate @ylecun's famous LeCake® -- we can take a picture of a cake, slice it, put one (or several) cherries on top, and even twist the stem to our liking -- I personally think it looks yummier than the original one :) .As far as I'm aware, this is a
2
5
31
Btw V-IRL ( VLN is such a natural setting to eval OOD behavior for multimodal agent: you can train the agent in one city, and then test it in a completely different environment. Same action space but completely different visual context to adapt to.
[3.2] 🗺️V-IRL consists two rules based on shifts of action orientation: {east, west, . } and {left, right, . }. Visual variants include training are characterized by different locations (e.g., train the agent to navigate in one place, and ask it to navigate in another).
0
9
32
Congrats! It finally feels like the AI version of a CERN paper now :) We should have more global/open initiatives of this kind. Next up, 5000 co-authors 😀?.
It was super fun working with @QuanVng and the Google team on building a robot model that works across robots. While the results are wonderful, I'm most excited about the data from several amazing labs being accessible on a common platform.
2
1
31
@giffmana Haha, I think this looks much better than my rushed deadline attempt. (If you're not sure what Lucas is referring to, this was the original one.)
3
1
31
Finally, visual search has been a core problem in cogsci / vision science for decades (e.g. pioneering work by @jeremymwolfe). Interestingly, when compared with human eye fixations, the LLM guided V* can attain an efficiency comparable to that of human visual search! (6/n)
2
1
29
@GoogleDeepMind @LFC So glad to see this as a kop, can it pull off a corner taken quickly though? ;).
1
0
24
A new efficient video captioner and benchmark. Now available open source too. Led by the amazing @re5e1f !.
(1/n) 📸Want to train a better video generation model? You need a better video captioner first! Check out AuroraCap! Trained on 20M+ high-quality data, it’s more efficient at inference. #lmms #videogen.🔗Homepage:
1
0
27
exactly. that’s the power of open science and knowledge sharing: we learn and gain confidence in what we find!.
PS: A couple other very nice papers came out during the making of this (MM1, Cambrian-1, Idefics2, . what an active field!), which reflect some of our findings - great, we can be more confident about those!.
1
2
25