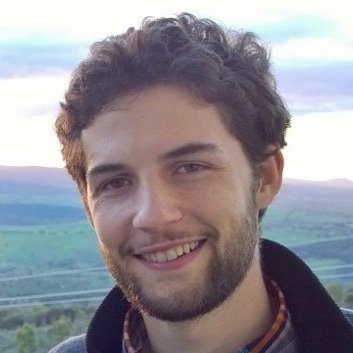
Tim Duignan
@TimothyDuignan
Followers
6K
Following
6K
Media
235
Statuses
4K
Modelling and simulation using quantum chemistry, stat mech and neural network potentials #compchem #theochem Researcher at Orbital Materials
Brisbane, Queensland
Joined February 2013
So I think I've found another pretty incredible example of the generalisability of neural network potentials: this is a problem I've been dreaming of tackling for a decade but never felt I had the the tools to get at until now: How do potassium ion channels work. 1/n. These
This is the most surprising and exciting result of my career: we were running simulations of NaCl with a neural network potential that implicitly accounts for the effect of the water, ie a continuum solvent model (trained on normal MD) when Junji noticed something strange: 1/n
10
56
377
RT @mmoderwell: I'm starting on a material doping study this week. In our rare-earth-free permanent magnet exploration, we've found some s….
0
0
0
Check it out:.
Imagine being able to run a full chemical simulation within minutes?. Watch below as Dr. @TimothyDuignan, one of our researchers, prompts our AI agent to run a full simulation of NaCl crystallization with just a single sentence prompt, in minutes. It’s the kind of task that would
2
1
26
RT @FrankNoeBerlin: After a 4-year journey, we are super happy to see this paper out in @NatureComms - @ElezKatarina et al: High-throughput….
nature.com
Nature Communications - Approaches making virtual and experimental screening more resource-efficient are vital for identifying effective inhibitors from a vast pool of potential drugs but remain...
0
36
0
Yeah so important to build these data sets.
@Robert_Palgrave @bryancsk personally, i'm interested in high-quality datasets for defects, interfaces, phase transitions, many-component systems, magnetic systems, systems subject to external fields. you could gain a lot from 100k-1M diverse samples at a high-level of theory in each of these spaces.
0
1
11
RT @marceldotsci: overall, it seems that we need more challenging benchmarks. or maybe LR behaviour is simply "not that complicated". we wi….
arxiv.org
Machine learning interatomic potentials trained on first-principles reference data are quickly becoming indispensable for computational physics, biology, and chemistry. Equivariant message-passing...
0
1
0
RT @marceldotsci: ✨preprint alert: "learning long-range representations w/ equivariant messages" in which we get into the fray of long-rang….
0
8
0
RT @TimothyDuignan: @Robert_Palgrave @jrib_ @bryancsk The evidence is increasing that training on quantum chemistry datasets produces model….
0
2
0
RT @j0hnparkhill: Every University and Academic should be spending at least 10% of their resources pondering, planning and reinventing for….
0
2
0
Yes I think of rational design of materials at the atomistic scale as a keystone problem: Once you unlock it the possibilities are endless and will set off a cascade of breakthroughs throughout the sciences and engineering.
30% of the global economy depends on advanced materials. Yet we still discover them the way we did 200 years ago: mix, heat, test, repeat. At Orbital, we use AI to design materials and because we’re fully verticalized, turn them into deployable hardware. Our CEO Jonathan
4
11
61
RT @jrib_: Two updates to share, one personal and one about open source which I'm very excited about!.Personal: My days in NYC are over. 4….
0
25
0
RT @SzilvasiGroup: Our contribution to the Athanassios Z. Panagiotopoulos Festschrift is now online. We show how to model complex aqueous….
pubs.acs.org
Understanding the structure and thermodynamics of solvated ions is essential for advancing applications in electrochemistry, water treatment, and energy storage. While ab initio molecular dynamics...
0
3
0
RT @CatAstro_Piyush: will start posting blogs about my journey as I learn through my PhD here :).
0
1
0
RT @nanophononics: We investigated the formation of graphite and diamond from liquid carbon under high pressure. There are some surprises. ….
nature.com
Nature Communications - Molecular simulations reveal how diamond and graphite crystallize from molten carbon. Following Ostwald’s step rule, the liquid’s low density drives metastable...
0
3
0
RT @OrbMaterials: A new evaluation shows our AI simulation model, Orb, stands out in many tests. 👏. In this paper (arXiv:2506.01860) by Bow….
0
3
0
RT @mmoderwell: Best not to lie to ourselves in materials discovery. The last thing you want is to have a good candidate material you can't….
0
5
0
RT @smnlssn: Time’s running out! Lead generative AI research for molecular dynamics in our lab AIMLeNS. - Scale our NeurIPS/ICLR-published….
0
7
0
RT @mmoderwell: ⚛️ Phase-diagram and energy-above-hull endpoint is live!. 🔎 This new endpoint compares your structure to other known materi….
0
5
0
RT @bwood_m: 🚀Exciting news! We are releasing new UMA-1.1 models (Small and Medium) today and the UMA paper is now on arxiv! . UMA represen….
0
28
0