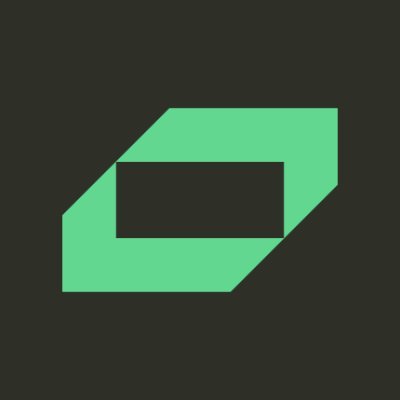
vespa.ai
@vespaengine
Followers
3K
Following
754
Media
27
Statuses
501
https://t.co/abkb8IjPSH - the open source platform for combining data and AI, online. Vectors/tensors, full-text, structured data; ML model inference at scale.
Joined September 2017
LangChain Webinar on retrieval for LLMs with @lateinteraction and our very own @jobergum in six hours. Save your spot at
1
6
31
Having trouble keeping up? . Guidebook to the State-of-the-Art Embeddings and Information Retrieval .by @aapo_tanskanen at @thoughworks is out today - a great resource to get up to date.
0
9
26
What the world need most now is more research on #covid19, faster. We've created to help with that. It lets researchers find research papers by combining text and structured search with exploring by semantic similarity using the scibert-nli model.
The @vespaengine team released based on the CORD-19 dataset released by the @allen_ai. Since everything is open-sourced, you can contribute to the project in multiple ways. ๐. #NLP #NLProc #SearchEngine #COVIDใผ19.
0
14
26
Follow this guide if you need to build a personal data app which .- costs about 5% of a traditional vector database.- contrary to those, finds all the user's data.- scales to millions (or billions) of users.
Hands-on RAG guide for personal data with @vespaengine and @llama_index ๐ . Featuring LLamaIndex retrievers, Vespa streaming mode for personal data, built-in embedders, multi-index RAG (federation + blend), hybrid search, and rank fusion strategies.
1
4
19
Recommender systems need to multiply very large sparse matrices. e-commerce platform @farfetch leverages Vespa's support for sparse and dense tensors + vector search to do this online in less then 100 milliseconds. ๐งต.
1
1
19
The latest Vespa newsletter is here to help you stay up to date on what's happening on the leading edge in RAG, IR and vector search:.- A new SPLADE embedder.- ONNX models with float16.- @cohere embedding model guides.- Support for an array of chunks with ColBERT.- And list of.
0
3
18
Very informative post on choosing Vespa for personalization using vector embeddings
Blog post on how โฆโฆ@vintedโฉ started using โฆ@vespaengineโฉ for recommendations
2
5
15
When you are doing nearest neighbor vector retrieval you are doing *search*, which is computation over data. The llm builders community is currently speedrunning the process of discovering what this takes. If you are reading this, congrats you're likely ahead of the curve :-).
Vector databases are not search engines. Reasoning over metadata is easily achievable with COT + @elastic , @vespaengine , or other search engines.
0
0
15
Vespa continues to empower developers who are building something real with vector embeddings. No custom code required to.- use any embedding model from @huggingface.- do multilingual embedding.- run embedders on GPUs.
1
0
13
Highlights of Vespa features released in June:. - Personal vector search with complete results, at 1/20 of the cost, with streaming.- MIPS/dot product ranking in ANN.- GPU Acceleration of embedding models.- Use any @huggingface embedder directly.
0
4
12
If you are looking for a comparison of Elasticsearch, Solr, and Vespa this is the video to watch.
Find out how our invited speakers compared #Elasticsearch #Solr and @vespaengine at the Haystack LIVE! Meetup - video now available at - thanks so much @anshumgupta @jobergum @joshdevins !.
0
2
11
If you are using vector embeddings, reading this post might be the most profitable ten minutes you'll ever spend.
Matryoshka ๐ค Binary vectors: Slash vector search costs with Vespa. We announce support for combining matryoshka and binary quantization in Vespaโs native hugging-face embedder and discuss how this slashes vector search costs.
0
1
10
April @vespaengine updates include performance and operability improvements: Top-K hits, smarter data migration and CloudWatch integration. Contributing to Vespa is now easier with the release of a CentOS 7 dev environment. #bigdata.
0
6
11
We're submitting some Vespa examples to relevance competitions. All of them:.- available as open source sample applications.- production ready, with < 100 ms latency. This first one is a simple WAND+GDBT baseline, beating all the other entries not using deep learning/embeddings.
Welcome @jobergum and @vespaengine to the fray with their first @MSMarcoAI document leaderboard submission! Awesome to see LTR effectiveness creeping up on the muppets.
1
3
10
Did you know you can now convert text to vector embeddings in documents and queries automatically in Vespa? We provide the wonderful SentencePiece algorithm by Taku@Google for this, but you can also plug in your own.
0
2
10
Finally, for all the Elastic developers out there who want to start creating modern search base applications, @atitaarora on understanding Vespa with a Lucene mindset
0
3
9
Testing ANN performance on Search a million vectors in 2 ms measured from the client.
From our performance factory page at @vespaengine HQ where we test Vespa performance for every build. This is testing Vespa's approximate nearest neighbor search on SIFT 1M This is an end to end benchmark including HTTP API. Pretty awesome if you ask me.
0
3
9
ColBERT v2 is the state of the art in information retrieval, and with this work you can actually run it economically, at any scale.
Announcing ColBERT in @vespaengine, enjoy!. - A new native Vespa ColBERT v2 embedder.- ColBERT token-level vector compression (32x).- Support for long context via Vespa mixed tensors.- Offload to disk .- Eval. Plus, it boasts the largest FAQ ever!๐
.
0
0
9
If you're in Bay Area come meet us at September 26. in San Francisco Thanks to @Amplitude_HQ for hosting!.
0
5
9
If you have it's not too late to make yourself a state of the art and infinitely scalable e-commerce site in time for Black Friday. @jobergum explains how in
0
9
9
For more on how to use reinforcement learning for recommendation see
A breath of fresh air: @vespaengine at #HaystackConf, signal to noise is through the roof! Reinforcement learning for comment ranking.
0
6
6
We also provide the same for *your* applications when running on Vespa Cloud.
@vespaengine has one of the best FOSS ci/cd set up I have seen out there. Pretty much everything is automated. This encourages contributors like me to contribute even more because the changes are so instant on prod.
0
0
7
Danswer (YC W24) on why they moved their RAG solution to Vespa. We hear this in so many private conversations right now.
@DanswerAI has been using @vespaengine as our search engine for a long time. In this blog, I outline the key benefits of using Vespa. Thanks @jonbratseth, @kraune and @jobergum for keeping Vespa open source and helping our users with Vespa questions!.
0
0
7
For those who want to contribute to this will be an easy way to get started.
Join us March 21 - 28, for Yahoo Hack Together, a virtual #opensource #hackathon! Learn more & register: #bigdata #design #devops #networksecurity
0
3
7
@farfetch The full post includes a detailed description of how to implement a scalable and low latency recommender system:
0
0
7
๐
The official 2024 Advent of Tensors ๐
. Follow this thread to get a challenge a day, win swag and become a tensor computation expert along the way.
Prepare to embark on a festive journey as we bring you the Advent of Tensors with 24 challenges and the chance to win @vespaengine swag! .
0
3
7
This is an unusually good comparison, although it leaves out what we see as most important: Ranking and inference capabilities. It can be solved elsewhere, but only if you don't need to perform at scale . .
Found this comprehensive spreadsheet on the key features of popular Vector Databases used to build out AI chatbot solutions. Spreadsheet: Key takeaways:. - The most well-rounded solutions include @weaviate_io, @vespaengine, and @elastic. - The top
0
0
7