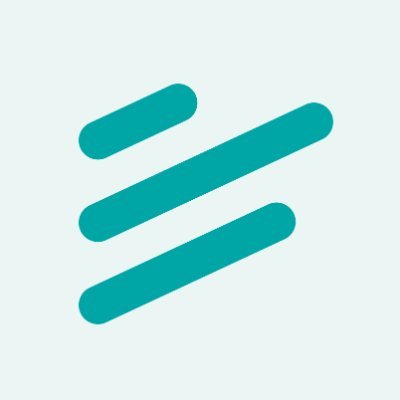
Epoch AI
@EpochAIResearch
Followers
24K
Following
417
Media
354
Statuses
1K
Investigating the trajectory of AI for the benefit of society.
Joined May 2022
@ansonwhho @ardenaberg Read @ardenaberg and @ansonwhho’s full analysis of a possible AI Manhattan Project
0
1
16
@ansonwhho @ardenaberg Nonetheless, the authors conclude that a meaningful acceleration due to nationalization would be unwise to write off.
1
2
15
@ansonwhho @ardenaberg All of this is far from inevitable, especially because of serial time bottlenecks and the need for strong political buy-in. e.g. government inefficiencies could substantially increase costs, reducing the size of the largest training run at Manhattan project-sized budgets.
1
1
11
@ansonwhho @ardenaberg This much compute in 2027 would require 7.4 GW, more than the average power usage of New York City. A Manhattan Project could likely support this by gathering already planned new capacity, especially given DPA authority.
1
1
14
@ansonwhho @ardenaberg With this much investment, the project could support a training run of 3e29 FLOP in 2027. This is roughly a 1000x increase relative to the largest models of today, and 2 years earlier than existing trends indicate.
1
2
21
@ansonwhho @ardenaberg Previous national projects at their peaks spent an equivalent fraction of GDP as $120B-$250B today. The authors find that such a budget could centralize most NVIDIA compute in the US.
2
1
20
@ansonwhho @ardenaberg A national AI project has become more and more of a possibility in the last year, with one as the top recommendation from a US-China congressional commission.
1
2
18
What would a Manhattan Project for AI look like?. @ansonwhho and @ardenaberg argue that if one reaches the scale of previous national projects, an AI Manhattan project could result in a ~1000x compute scaleup by 2027.
9
36
206