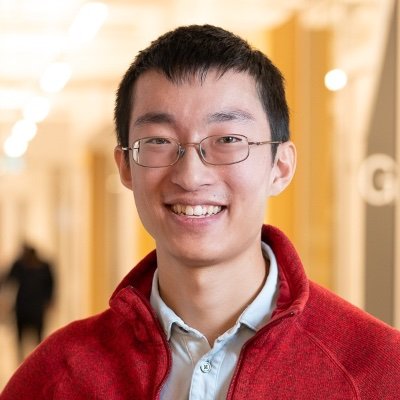
Alex Tong
@AlexanderTong7
Followers
2K
Following
319
Media
10
Statuses
164
Postdoc at Mila studying cell dynamics with Yoshua Bengio. I work on generative modeling and apply this to cells and proteins.
Joined May 2017
Excited to share our new work on Meta Flow Matching (MFM)! 🎉 MFM helps improve the generalization of personalized treatment response predictions by conditioning on learned features of the initial distribution. Work led by @lazar_atan and @NZhang211.
🚀Introducing — Meta Flow Matching (MFM) 🚀. Imagine predicting patient-specific treatment responses for unseen cases or building generative models that adapt across different measures. MFM makes this a reality. 📰Paper: 💻Code:
0
7
79
Excited to share our latest work on Metric Flow Matching (MFM)! Loved working with this team. Amazing to see how far we've come computationally since I started working in this field.
If data lives on a manifold, how do we design meaningful interpolations between marginals? We present Metric Flow Matching (MFM)…. @PPotaptchik @TeoReu @leoeleoleo1 @AlexanderTong7 @mmbronstein @bose_joey @Francesco_dgv . 🔗Dive in here: 🧵 (1/12)
0
11
73
Happy to introduce our work on DEM which builds on incredible work on FAB by @SilkyDogfish, Vincent Stamper, @GregorSimms @bschoelkopf and @jmhernandez233 and Equivariant FM by @leonklein26 Andreas Krämer and @FrankNoeBerlin.
No data? No problem🚀! Introducing iDEM a new method to sample from Boltzmann distributions. arXiv: co-led with @tara_aksa, @jarridrb. and amazing collaborators: @sarthmit @PabloLemosP @ChengHaoLiu1 @MarcinSendera Siamak Ravanbaksh Yoshua Bengio
3
10
70
Excited to share our latest work on discrete diffusion models and the DDPP objective. A new approach for simulation-free fine-tuning. Check it out if you’re interested!.
🚨Discrete diffusion models are the next big thing. But guess what? RLHF-style fine-tuning and guidance for them aren’t the answer! ❌. What does work? 🚀The simulation-free DDPP objective. Check out our latest work for all the details. 👇 a 🧵. Arxiv:
0
9
49
Check out this extension of DynGFN (with @lazar_atan and @jasonhartford) which improves scalability with a more efficient parameterization. Reducing trajectory lengths from n^2 --> n.
Curious about how to discover causal interactions among many genes in a cell with uncertainty estimation?.@TrangNguyen2399 @AlexanderTong7 . Check our new work :
0
5
48
Happening in ~2 hours! Code available here:
Diffusion models are dead - long live joint conditional flow matching! 🙃.Tomorrow @AlexanderTong7 presents his "Improving and generalizing flow-based generative models with minibatch optimal transport" On Zoom 11am EDT / 3pm UTC:
0
8
45
Had a great time working with this team. See our first work on flow matching for protein generation. More to come!.
What's better than Diffusion for protein backbone generation?.(Stochastic) OT Flow Matching over SE(3)! link: New paper w/ @tara_aksa @FatrasKilian @Guillaume_hu @jarridrb @ChengHaoLiu1, @andreic_nica @MaksymKorablyov @mmbronstein @AlexanderTong7 .1/5🧵
0
5
38
Very cool application of Flow matching! Multi-Ligand binder design by learning flows from a prior with separated ligands and target.
New paper!🤗."Harmonic Self-Conditioned Flow Matching for Multi-Ligand Docking and Binding Site Design" Generating pockets to bind small molecules has applications like designing antidotes that sequester toxins or as a first step toward Enzyme design.1/11
1
4
37
Looking forward to presenting this work with @Guillaume_hu tomorrow!.
This week, 8/06 @ 4 pm ET, we'll have @AlexanderTong7 present . Sequence-Augmented SE (3)-Flow Matching For Conditional Protein Backbone Generation. Read about FoldFlow . FF2: FF1: Sign up to receive the Zoom link via email!.
0
6
36
Checkout our new protein model @DreamFoldAI thread by first author @Guillaume_hu. Great work by the team and proud of our results!.
Introducing FoldFlow-2, a new SOTA sequence-conditioned protein generative model!. work w/ @DreamFoldAI James V @FatrasKilian Eric TL @PabloLemosP @riashatislam @ChengHaoLiu1 @jarridrb @tara_aksa @mmbronstein @AlexanderTong7 @bose_joey. Arxiv: 1/8 🧵
0
3
33
@KrishnaswamyLab @CellCellPress @christophertape @Maria_Ramos_Z Optimal transport is incredibly useful in single-cell analysis, but how do you handle controls? @christophertape already has a great thread on the biological impact, so in this thread I’ll focus on the ML and computational aspects. (1/n)
1
5
25
I wholeheartedly agree😀! Great working with @christophertape and @Maria_Ramos_Z on this!.
It has been such an enormous privilege to work with @KrishnaswamyLab and @AlexanderTong7 on this project. Alex is currently on the faculty job market and anyone who has any sense should hire him immediately.
0
0
14
Great tutorial on Trellis created by @Maria_Ramos_Z which shows how to combine hierarchical clustering and optimal transport for compare a large number of single-cell distributions.
Here it is! We have now created a how-to tutorial to understand and use Trellis, a tool for hierarchical clustering that enables the fast calculation of distances across thousands of distributions containing single-cell information:
0
1
13
Awesome blog posts on the state of the field by @michael_galkin and @mmbronstein!.
📣Two new blog posts - a comprehensive review of Graph and Geometric ML in 2023 with predictions for 2024. Together with @mmbronstein, we asked 30 academic and industrial experts about the most important things happened in their areas and open challenges to be solved. 🧵 1/n.
0
1
12
Great working with this incredible team!.The most recent version (with updated results) is available on the open review site here:
Happy to share that FoldFlow, the first Flow Matching generative model for Protein backbone generation got accepted to #ICLR2024 as a Spotlight with scores 8/8/8/8! Thanks to the reviewers for the feedback and ofc all my incredible co-authors for pulling this fun paper off🚀!.
0
0
11
Hope to see you there! TL;DR GflowNets for Bayesian (Dynamic) Gene Regulatory Network discovery.
I will be at #NeurIPS2023 this week! I am excited to be presenting DynGFN during the main conference. Looking forward to seeing everyone there! . Poster session 4, Wed, Dec 13th 5 - 7 pm CST. @VectorInst @Mila_Quebec @valence_ai.
0
1
9
Can't recommend working with @christophertape and lab enough! An awesome opportunity.
1 week left to apply for our computational PhD project 'Single-cell Signalling Analysis of Stem Cell Polarisation in Cancer'. 4-years, fully funded, co-supervised across @uclcancer @crick with a placement at @Yale . Pls RT!.
0
1
8
@jonkhler @SilkyDogfish @GregorSimms @bschoelkopf @jmhernandez233 @leonklein26 @FrankNoeBerlin @smnlssn @__kraemer__ Thanks Jonas for your thoughts! I believe your understanding is correct. When we test on the 55 particle Lennard-Jones system we get forces on the order of 10^30. In practice we clip and it seems to work fairly well. Definitely interested in how well it extends to proteins :).
1
0
6
@KrishnaswamyLab @CellCellPress @christophertape @Maria_Ramos_Z Thank you for reading!.Code: Tutorial: Raw data: Preprocessed data: (10/n).
0
1
5
@alexechu_ @bose_joey @json_yim Hi @alexechu_, I was also curious about this. (1) It turns out that FM and diffusion have slightly different conditional paths as shown in Figure 3 of (2) the conditionals for the VE ODE are an optimal transport map for a linear noise schedule.
1
0
4
Great feature by @kevanchu and @dow_lab on our work on Trellis studying the Tumor microenvironment in PDOs.
Check out this Cancer Research feature on our recent work on PDOs and Trellis with @christophertape @AlexanderTong7 @Maria_Ramos_Z !
0
0
4
@KrishnaswamyLab @CellCellPress @christophertape @Maria_Ramos_Z I’m excited to see what types of methods can be developed using this data. High-level summary, 25m cells, 44 features in a tensor of 10 patients, 11 drug combinations, 3 co-cultures states, with 3 replicates, which presents many opportunities for splitting and validation. (9/n).
1
0
3
@KrishnaswamyLab @CellCellPress @christophertape @Maria_Ramos_Z Trellis embeds distributions to vectors where the Wasserstein distance between distributions is equivalent to the L^1 distance between vectors. On a tree with hierarchical clustering, we compute the cell abundances in each node of the tree weighted by the edge weight. (7/n).
1
0
2
@KrishnaswamyLab @CellCellPress @christophertape @Maria_Ramos_Z The L^1 distance between these vectors is now the Wasserstein distance. This means that when used to create manifold-based embeddings between distributions which only require nearest neighbor distributions, Trellis scales log-linearly in the number of cells and samples. (8/n)
1
0
2
@yuanzhi_zhu @iScienceLuvr Yes! Cool to see what we call the minibatch-OT trick also works in these settings!. Some works that may interest the authors (@Yiheng_Li_Cal @Chenfeng_X) that also use this trick:.OT-CFM Multisample-FM A-OT
1
0
1
@KrishnaswamyLab @CellCellPress @christophertape @Maria_Ramos_Z This means that we can greatly reduce technical variation by comparing how treatments affect the cell distribution within the same plate. (5/n).
1
0
2
@KrishnaswamyLab @CellCellPress @christophertape @Maria_Ramos_Z Since this dataset is so large, it was collected using many individual experiments. This leads to technical batch effects. What saves us here is that each experiment has its own controls. (3/n)
1
0
2
@KrishnaswamyLab @CellCellPress @christophertape @Maria_Ramos_Z Single cell datasets are getting larger and larger. Heren @maria_ramos_z profiled >25 million cells in 3,360 separate samples spanning patient, drug, and microenvironment specific effects. At this scale it’s difficult to understand the data let alone draw insights. (2/n).
1
0
2
@KrishnaswamyLab @CellCellPress @christophertape @Maria_Ramos_Z Since this dataset is so large, it was collected using many individual experiments. This leads to technical batch effects which can drown out subtle biological signals. What saves us here is that each individual experiment has its own controls. (4/n)
1
0
2
@KrishnaswamyLab @CellCellPress @christophertape @Maria_Ramos_Z We developed Trellis as an extremely fast and interpretable method to understand how a treatment changes the observed cell states. Trellis is able to measure the changes in changes of cell state distributions by how much work it takes to transport over a cell state tree. (6/n)
1
0
2